Neural networks are used for recognizing pictures, understanding natural language with good accuracy, automate driving vehicles and a lot of other applications follow. But still, neural networks need human supervision to learn. Usually, a network needs labeled examples to learn effectively. While it’s also possible to learn from unlabeled data, this had typically not worked very well.
GANs was proposed by Ian Goodfellow (currently a staff research scientist at Google Brain). By applying game theory, he devised a way for a machine-learning system to effectively teach itself about how the world works. This ability could help make computers smarter by sidestepping the need to feed them painstakingly labeled training data. Generative Adversarial Networks (GANs) are neural networks that are trained in an adversarial manner to generate data mimicking some distribution.
To explain it in simpler terms:
If you want to get better at something, say chess; what would you do? You would compete with an opponent better than you. Then you would analyze what you did wrong, what he/she did right, and think on what could you do to beat him/her in the next game.
You would repeat this step until you defeat the opponent. This concept can be incorporated to build better models. So simply, for getting a powerful hero (viz generator), we need a more powerful opponent (viz discriminator)!
To understand this deeply, first, you’ll have to understand what a generative and a discriminative model is.
In machine learning, the two main classes of models
- Discriminative – A discriminative model is one that discriminates between two (or more) different classes of data – for example, a convolutional neural network that is trained to output 1 given an image of a human face and 0 otherwise.
- Generative – A generative model, on the other hand, doesn’t know anything about classes of data. Instead, its purpose is to generate new data which fits the distribution of the training data – for example, a Gaussian Mixture Model is a generative model which, after trained on a set of points, is able to generate new random points which more-or-less fit the distribution of the training data (assuming a GMM is able to mimic the data well).
The generative network’s training objective is to increase the error rate of the discriminative network (i.e., “fool” the discriminator network by producing novel synthesized instances that appear to have come from the true data distribution). In practice, a known dataset serves as the initial training data for the discriminator. Training the discriminator involves presenting it with samples from the dataset until it reaches some level of accuracy. Typically the generator is seeded with a randomized input that is sampled from a predefined latent space (e.g. a multivariate normal distribution). Thereafter, samples synthesized by the generator are evaluated by the discriminator. Backpropagation is applied in both networks so that the generator produces better images, while the discriminator becomes more skilled at flagging synthetic images. The generator is typically a deconvolutional neural network, and the discriminator is a convolutional neural network.
Working of GANs explained:
So as we saw, there are two components in GANs
- Generator Neural Network
- Discriminator Neural Network
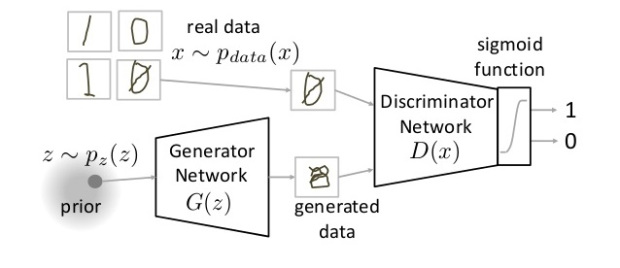
The Generator Network takes a random input and tries to generate a sample of data. In the above image, we can see that generator G(z) takes an input z from p(z), where z is a sample from probability distribution p(z). It then generates a data which is then fed into a discriminator network D(x). The task of Discriminator Network is to take input either from the real data or from the generator and try to predict whether the input is real or generated. It takes an input x from pdata(x) where pdata(x) is our real data distribution. D(x) then solves a binary classification problem using sigmoid function giving output in the range 0 to 1.
Defining the notations used:
Pdata(x) -> the distribution of real data
X -> sample from pdata(x)
P(z) -> distribution of generator
Z -> sample from p(z)
G(z) -> Generator Network
D(x) -> Discriminator Network
Steps to train a GAN:
Step 1: Define the problem. Do you want to generate fake images or fake texts? Here you should completely define the problem and collect the data for it.
Step 2: Define architecture of GAN. Define how your GAN should look like. Should both your generator and discriminator be multi layer perceptrons or convolutional neural networks? This step will depend on what problem you are trying to solve.
Step 3: Train Discriminator on real data for (n) epochs. Get the data you want to generate fake on and train the discriminator to correctly predict them as real. Here value (n) can be any natural number between 1 and infinity.
Step 4: Generate fake inputs for generator and train discriminator on fake data. Get generated data and let the discriminator correctly predict them as fake.
Step 5: Train generator with the output of discriminator. Now when the discriminator is trained, you can get its predictions and use it as an objective for training the generator. Train the generator to fool the discriminator.
Step 6: Repeat step 3 to step 5 for a few epochs.
Step 7: Check if the fake data manually if it seems legit. If it seems appropriate, stop training, else go to step 3. This is a bit of a manual task, as hand evaluation of the data is the best way to check the fakeness. When this step is over, you can evaluate whether the GAN is performing well enough.
The dueling-neural-network approach has vastly improved learning from unlabeled data. GANs can already perform some dazzling tricks. By internalizing the characteristics of a collection of photos, for example, a GAN can improve the resolution of a pixelated image. It can also dream up realistic fake photos, or apply a particular artistic style to an image. “You can think of generative models as giving artificial intelligence a form of imagination,” Goodfellow says.
(Sources: Wikipedia, MitTechReview, AnalyticsVidhya)